Technological development When "artificial intelligence" and the aviation industry are combined, everyone's reaction may be drones. But this is just the tip of the iceberg. Aircraft manufacturers and airlines have invested a lot of resources in artificial intelligence technology, actively exploring application scenarios, from the cockpit to the customer experience, almost all over the industry.
For many years, automated systems have been part of commercial aviation. Thanks to the use of "remote control driving" and automatic flight systems, machine learning and artificial intelligence technologies have also become "crew members." These systems are gradually able to play the role of co-pilot instead of simply reducing the workload of the pilot.
For example, a system originally developed for the safety of unmanned aerial vehicles (UAVs), such as broadcast-based automatic correlation surveillance (ADS-B) for traffic environment awareness, has been moved to the cockpit. In order to meet the need to compensate for driving control characteristics, people have developed a new system similar to the Maneuvering Characteristics Enhancement System (MCAS) to improve safety. These systems can automatically adjust the flight control surface using sensor data according to flight conditions.
However, the quality of machine learning systems depends on the data they get. Few people understand the risks inherent in allowing machine learning or artificial intelligence to take over human work in high-risk environments.
The recent Lion Air 610 crash is still under investigation. However, judging from the details disclosed so far, the aircraft has too much control over to the automatic system, there is a great risk. However, catastrophic aviation accidents rarely occur due to a single error (this time is no exception), MCAS sensor failure, maintenance fails to fully solve the problem, pilots are not fully trained and do not fully understand the functions and usage of MCAS Cause an accident.
The painful lesson learned from 189 lives is that the aviation industry must integrate data quality, attention and development of machine learning and artificial intelligence systems into the safety culture. Since machine learning and artificial intelligence have changed the role of pilots, we should also refer to similar roles and conduct a comprehensive test of these technologies: at least to achieve the same level, it is considered to be capable of the job.
Beyond autonomous driving
The Airbus A350 XWB aircraft exhibited during the 2015 Dubai Air Show has more than 50,000 sensors, and the daily flight and performance data collected totals more than 2.5TB.
Major aircraft manufacturers such as Airbus have gradually entered the AI ​​stage. According to Airbus Vice President AI Adam Bonnifield, the company is committed to developing these technologies for a long time.
"Because of our industry background and the experience we have accumulated in solving problems with autonomous systems in the past, we are not new to these technologies," he told us.
This modern airliner has a lot of data for machine learning to mine: Airbus (Airbus) launched the twin-engine wide-body aircraft A350 XWB in 2015 with about 50,000 sensors and can collect 2.5TB of data every day. Artificial intelligence can use this data in many ways.
Airbus is studying how to reduce the pilot's cognitive load (and the resulting cognitive fatigue) and reduce the number of cockpit pilots, so that the crew can invest more time to deal with the overall strategy and flight tasks, rather than spend a lot of time in All minor issues.
Bonnifield explained that although many people think that autopilot in an aircraft is "binary": automatic or not, he does not think so: "It is more like a spectrum," he said. "We try to solve it with artificial intelligence. Some minor problems during the flight. "
For example, a runway overrun protection option on Airbus aircraft. ROPS software is responsible for calculating the approach speed and weight of the aircraft. It compares the calculated physical model with the known runway length and real-time weather. If an unsafe condition is detected, it will broadcast "Runway too short!" ROPS also You can calculate a good taxi slope or trajectory for landing and help with other operations such as taxiing, takeoff, and flight.
Another key area of ​​AI for Airbus is the construction of self-driving cars and air taxis to provide transportation services for urban residents. When a pilot loses consciousness due to a drop in cabin pressure, artificial intelligence may take over the aircraft. Under high pressure, assuming that the data is correct, artificial intelligence can integrate various factors more quickly, make more reasonable decisions, and increase safety.
Make communication easier
Air traffic controller at the London Regional Control Center LACC. The thick English accent in the noisy communication channel is the real test of the speech recognition system.
Air Traffic Control (ATC) communication is critical for all flights.
In European airspace, many dialogues are heavily accented, making it difficult for pilots and controllers to understand each other. When the pilot cannot see the situation outside the cockpit, he needs to listen to the tail / flight number under instrument meteorological conditions (IMC) to obtain information such as direction indications and traffic alerts. Airbus intends to use artificial intelligence to solve the speech recognition problem, which is also part of its AI Gym competition. In the AI ​​Gym program, Airbus seeks external cooperation to help develop breakthrough artificial intelligence systems.
The clarity of the air traffic dialogue is a very difficult task for machine learning, because ATC audio is very noisy, and the conversation is fast, full of "domain-specific vocabulary." AI Gym hopes to provide complete transcription of ATC audio and extract aircraft call signals from the audio for call tracking and alarming.
In October 2018, the competition ended, and Airbus had already begun to convert the research results into products. AI Gym allows Airbus to leverage external expertise to explore many other potential uses of artificial intelligence.
"We have many problems and interesting cases that need to be explored and solved." Bonnifield said. Part of the reason is that this technology is relatively new and is at a very immature stage. There are many experiments and some great open source technologies.
With the help of this project, Airbus is collaborating with "ordinary suspects" (the name of the American movie, which is used here as a metaphor for the privacy of cooperation because of the confidentiality agreement). This way of cooperation has the advantage: even if it fails, it will not be known to the world. Although we also expect these mysterious partners to provide high-performance solutions, Bonnifield found that most of the good solutions come from small and micro startups.
Only a small number of research teams can often provide good solutions. Bonnifield said he believes this may be unique in the field of AI. The big challenge facing Airbus is how to bring these small teams at the forefront of innovation together and provide them with a simple way to collaborate.
This requires Airbus to change the way it cooperates with the outside world. "Some startups have even done RFPs before," Bonnifield explained.
Landing to business
In terms of flight safety, airlines rely heavily on equipment manufacturers (such as Airbus and Boeing). However, airlines also need machine learning and artificial intelligence to assist in internal management—to streamline ground operations and create a better customer experience by achieving a “seamless journey†as much as possible.
United is investing in all available new technologies, using machine learning to collect back-end data from customers, maintenance logs, employee duty logs, and progressive data in flight to improve business overall. Praveen Sharma, vice president of digital products and analysis at United Airlines.
In September, United and Palantir announced a long-term partnership, using Palantir Foundry as the airline ’s central platform to accelerate the enterprise-level data plan between key internal business units.
"The real challenge is ... integrating the massive amounts of data from different platforms across the company's departments into one platform ... We can use this platform to build machine learning and artificial intelligence models." Sharma said that in the past year, to achieve this , The two companies launched a series of research projects.
Palantir partnered with Airbus to create Skywise, an aerospace data analysis platform that Airbus provides ordering services for small airlines, including maintenance tools to help reduce flight accidents. GE also tried to convert aircraft sensor data into a machine learning-based service to drive predictive maintenance of the company's jet engines.
United Airlines and its regional airlines United Express operate approximately 4,600 flights daily to 357 airports on five continents. Last year, the two companies operated more than 1.6 million flights and carried more than 148 million passengers. When unforeseen maintenance problems or other operational problems occur, United will use machine learning to collaboratively replace the aircraft. This is not as simple as people think; the system must consider all the variables required to allocate crew members (such as rest time and crew aircraft certification), aircraft fuel and operating restrictions, and aircraft seat capacity.
"These complex decisions often have to be made within 25 minutes based on the limited data available at the time," Sharma explained.
Not just maintenance
United ’s use of machine learning and artificial intelligence goes far beyond managing maintenance and aircraft schedules. It can also utilize customer data. Based on the interactive data of each passenger, United Airlines uses artificial intelligence and machine learning to optimize the customer experience—adjusting ticket prices to match passenger data.
United's machine learning algorithm can obtain 150 different customer and flight data points and decide in real time which product should be displayed to the customer at the point of purchase or registration. The engine considers factors such as passengers' previous purchases, preferences, destinations, and activities.
The interaction through the real-time decision engine began in 2014. The engine provides customers with various product options to improve the travel experience, such as flight selection, seat upgrades, mileage purchase or priority check-in.
Sharma said that United used a prediction model based on Bayesian inference. "It not only determines the content provided," Sharma explained, "but also determines the image and slogan displayed in front of customers."
Sharma said that the application of machine learning is paying off. According to the measurement results collected by United, customers no longer have to look for what they want to buy or want to experience.
Other airlines are also using artificial intelligence in other ways to reduce travel discomfort (and reduce the workload of airline employees). Face recognition technology is now appearing in terminals to help airport passengers expedite check-in. Most face recognition algorithms are based on deep learning, which is part of machine learning. Delta Air Lines is an airline that implements this process, and the company estimates that the move shortens passenger boarding time by nearly 10 minutes. The system is currently used for check-in and baggage check-in for international flights. Delta expects to expand it to domestic flights next year.
Disaster prevention
One of the important uses of artificial intelligence may be to identify security risks before disasters occur. For example, the Lion Air Flight 610 crash and the failure of the automatic control system may indicate a major safety issue.
The NASA Ames Research Center in Silicon Valley is currently engaged in aviation-related artificial intelligence research. One project focuses on identifying "abnormal operations" in commercial aviation event data, and these data may indicate potentially greater problems. This is precisely Nikunj Oza's main research area. Nikunj Oza is a computer scientist in charge of the intelligent systems department (ie Code TI) of the NASA Ames Research Center. Because the safety record of commercial aviation is very good-much better than driving, it is much more difficult to identify anomalies that may have safety problems.
NASA has initially developed some algorithms, which are mainly used for abnormal situation monitoring and identification of accident precursors, and has begun to receive relevant feedback from experts. Currently, NASA is developing a system for aircraft data security analysis-specifically for the Federal Aviation Administration data analysis partner Mitre.
Mitre is a federally funded research and development center that is implementing the "Aviation Safety Information Analysis and Sharing (ASIAS) Program," a data alliance-NASA, the Federal Aviation Administration, the National Transportation Safety Board, aircraft manufacturers, and more than 50 airlines. Share secure data between companies. The airline uploads a subset of flight record data to Mitre, and Mitre analyzes and provides feedback on potential problems. (Data is secretly shared by airlines.)
The data analysis method being developed by Ames Research Center hopes to use artificial intelligence to discover abnormal patterns in flight data in time (this may indicate that the aircraft has systemic problems).
"You want to find problems and find solutions as soon as possible to prevent this from happening again," Oza explained. So far, artificial intelligence has not completely replaced humans in aviation, and the relationship between artificial intelligence and human experts has proved to be complementary. -A partnership that can save lives.
Skylight opal acrylic properties and advantages
- - High gloss finish
- - Half the weight of glass
- - Easy to work and use
- - Good impact resistance
- - Perfect for use in Skylights, Bathroom, Kitchen and Room Dividers
- - Can be back lit with LED's and Light
- - Obscures and evenly diffuses light
- - Obscures and maintains privacy behind panel
- - Good electrical properties
- - High material stability and dimensional accuracy
- - Food and medical safe
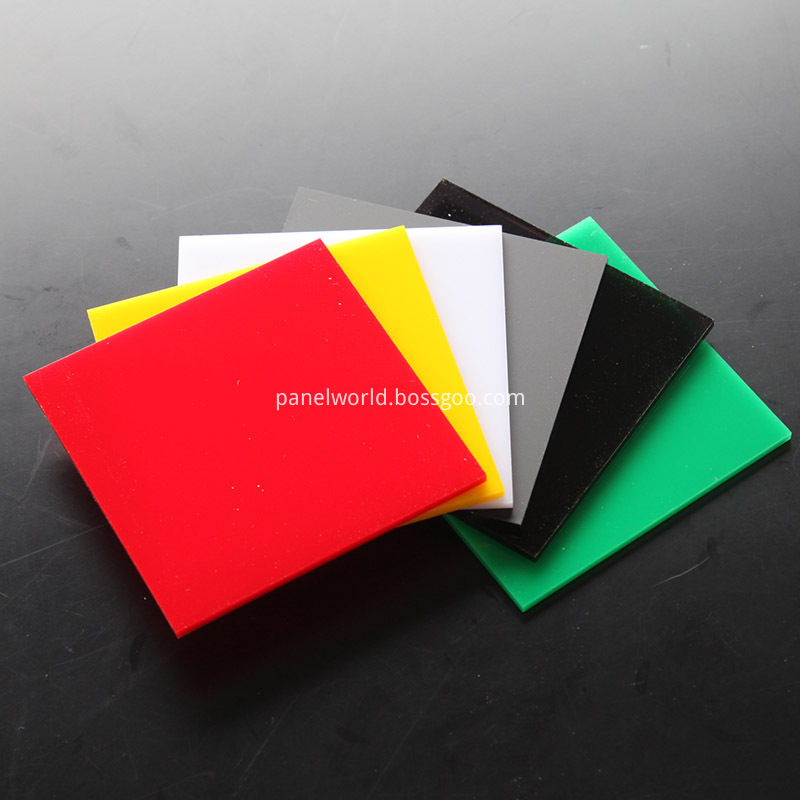
Skylight Opal Acrylic Plexiglass Sheet
Skylight opal acrylic sheets,Skylight sheets decorative,Skylight opal acrylic,Translucent skylight sheets
Aluminum sheet,Aluminum Composite Panel,Acrylic Sheets,PVC Expanded Plastic Sheet,Polypropylene Hollow Sheets , https://www.decoratepanel.com